Neel Nanda on mechanistic interpretability, superposition and grokking
The Inside View - En podkast av Michaël Trazzi
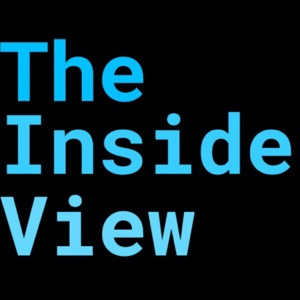
Kategorier:
Neel Nanda is a researcher at Google DeepMind working on mechanistic interpretability. He is also known for his YouTube channel where he explains what is going on inside of neural networks to a large audience. In this conversation, we discuss what is mechanistic interpretability, how Neel got into it, his research methodology, his advice for people who want to get started, but also papers around superposition, toy models of universality and grokking, among other things. Youtube: https://youtu.be/cVBGjhN4-1g Transcript: https://theinsideview.ai/neel OUTLINE (00:00) Intro (00:57) Why Neel Started Doing Walkthroughs Of Papers On Youtube (07:59) Induction Heads, Or Why Nanda Comes After Neel (12:19) Detecting Induction Heads In Basically Every Model (14:35) How Neel Got Into Mechanistic Interpretability (16:22) Neel's Journey Into Alignment (22:09) Enjoying Mechanistic Interpretability And Being Good At It Are The Main Multipliers (24:49) How Is AI Alignment Work At DeepMind? (25:46) Scalable Oversight (28:30) Most Ambitious Degree Of Interpretability With Current Transformer Architectures (31:05) To Understand Neel's Methodology, Watch The Research Walkthroughs (32:23) Three Modes Of Research: Confirming, Red Teaming And Gaining Surface Area (34:58) You Can Be Both Hypothesis Driven And Capable Of Being Surprised (36:51) You Need To Be Able To Generate Multiple Hypothesis Before Getting Started (37:55) All the theory is bullshit without empirical evidence and it's overall dignified to make the mechanistic interpretability bet (40:11) Mechanistic interpretability is alien neuroscience for truth seeking biologists in a world of math (42:12) Actually, Othello-GPT Has A Linear Emergent World Representation (45:08) You Need To Use Simple Probes That Don't Do Any Computation To Prove The Model Actually Knows Something (47:29) The Mechanistic Interpretability Researcher Mindset (49:49) The Algorithms Learned By Models Might Or Might Not Be Universal (51:49) On The Importance Of Being Truth Seeking And Skeptical (54:18) The Linear Representation Hypothesis: Linear Representations Are The Right Abstractions (00:57:26) Superposition Is How Models Compress Information (01:00:15) The Polysemanticity Problem: Neurons Are Not Meaningful (01:05:42) Superposition and Interference are at the Frontier of the Field of Mechanistic Interpretability (01:07:33) Finding Neurons in a Haystack: Superposition Through De-Tokenization And Compound Word Detectors (01:09:03) Not Being Able to Be Both Blood Pressure and Social Security Number at the Same Time Is Prime Real Estate for Superposition (01:15:02) The Two Differences Of Superposition: Computational And Representational (01:18:07) Toy Models Of Superposition (01:25:39) How Mentoring Nine People at Once Through SERI MATS Helped Neel's Research (01:31:25) The Backstory Behind Toy Models of Universality (01:35:19) From Modular Addition To Permutation Groups (01:38:52) The Model Needs To Learn Modular Addition On A Finite Number Of Token Inputs (01:41:54) Why Is The Paper Called Toy Model Of Universality (01:46:16) Progress Measures For Grokking Via Mechanistic Interpretability, Circuit Formation (01:52:45) Getting Started In Mechanistic Interpretability And Which WalkthroughS To Start With (01:56:15) Why Does Mechanistic Interpretability Matter From an Alignment Perspective (01:58:41) How Detection Deception With Mechanistic Interpretability Compares to Collin Burns' Work (02:01:20) Final Words From Neel