Building a Risk-Scoring Model for Applications: Initial Algorithm and the Underlying Data Elements
Inside AppSec - En podkast av Contrast Security
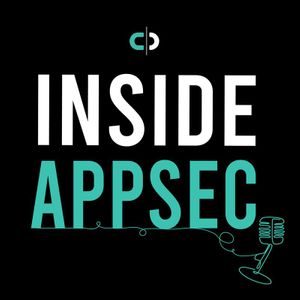
Kategorier:
Most risk-scoring models for applications are too simplistic, lacking the breadth of data points needed to provide an accurate risk index. A few open-source projects attempt to build application risk models that are sophisticated enough to account for all of the data and associated nuances needed to pinpoint a risk score that is accurate and meaningful. The problem is that they are too complex to easily implement and manage in an ongoing basis. In response, Contrast Security recently released a RiskScore (Beta V.5) based on an algorithmic risk model that accounts for all of the relevant data points that is also simple to use and manage. This Inside AppSec Podcast interview with Contrast CTO and Co-Founder Jeff Williams, CISO David Lindner, and Sr. Data Analyst and Data Scientist Katharine Watson explores the reasons Contrast developed an algorithmic RiskScore, why and how it plans to release it as an open-source project, how organizations can contribute to it and leverage it, and what the results resemble when it is applied to vulnerability types using Contrast Labs’ application vulnerability and attack data.